Yubo: a Secure Social Network
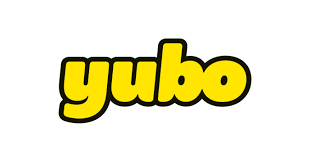
Yubo app: What is it and what does it do?
Yubo is a secure social network that allows users to connect with friends and strangers from around the world. Yubo makes it easy to find new people to chat with, and allows users to share their interests and experiences with others. Yubo is available for free on the App Store and Google Play.
The dangers of the Yubo app
When it comes to social media, there are a lot of great platforms out there that can connect you with friends and family all over the world. However, there are also some social media platforms that can be dangerous, especially for kids and teens. One of these platforms is Yubo, which is a social media app that is becoming increasingly popular with young people.
Yubo is a social media platform that is very similar to Snapchat and Instagram. Users can create a profile, add friends, and share photos and videos. However, one of the dangers of Yubo is that it does not have any age restriction, which means that anyone can join and use the platform. This can be very dangerous for kids and teens, who are more likely to be targeted by predators and cyberbullies.
Another danger of Yubo is that it allows users to share their location with other users. This can be very dangerous, especially for younger users, as it can put them at risk of being kidnapped or abducted.
Finally, Yubo also has a feature called “Live Streaming” which allows users to stream live video of themselves to other users. This can be very dangerous, as it can put users at risk of being watched by predators or cyberbullies.
If you are a parent of a child who uses Yubo, or if you are considering signing up for the platform, it is important to be aware of the dangers. You should talk to your kids about the dangers of sharing their location and personal information online, and you should also monitor their activity on the platform.
Yubo app: Why parents should be concerned
Yubo is a social media app that allows users to create and share content, as well as connect with other users. The app has been popular with teenagers and young adults, but has come under fire from parents and experts for its potential to be used for cyberbullying and other harmful behavior.
Here are three reasons why parents should be concerned about the Yubo app:
1. The app can be used for cyberbullying.
Yubo has been criticized for its potential to be used for cyberbullying. The app allows users to share photos and videos, as well as send messages to other users. This can be used to bully and harass other users.
2. The app can be used to share inappropriate content.
Yubo has been criticized for its potential to be used to share inappropriate content. The app allows users to share photos and videos, as well as send messages to other users. This can be used to share inappropriate content, such as nudity or violence.
3. The app can be used to groom children.
Yubo has been criticized for its potential to be used to groom children. The app allows users to share photos and videos, as well as send messages to other users. This can be used to groom children for sexual exploitation.
Yubo app: How to keep your child safe
As a parent, it’s important to be aware of the different ways you can help keep your child safe online. One way to do this is to monitor their activity on social media apps. Yubo is a social media app that allows users to connect with others based on their interests. It’s important to be aware of the potential risks associated with any social media app, and Yubo is no exception. Here are four tips to help you keep your child safe on Yubo:
1. Monitor their activity: Be sure to check in with your child regularly to see what they’re doing on Yubo. Pay attention to who they’re connecting with and what kinds of content they’re sharing. If you see anything that makes you concerned, reach out to them to discuss it.
2. Set up privacy controls: Yubo has privacy settings that you can adjust to help control who can see your child’s profile and what kind of information they can share. Be sure to go over these settings with your child and help them understand how to use them.
3. Teach them about online safety: It’s important to talk to your child about online safety in general, even if they’re not using Yubo. Help them understand the importance of being careful about what they share online and who they connect with.
4. Keep the lines of communication open: One of the most important things you can do is to keep the lines of communication open with your child. Let them know that they can come to you with any concerns or questions they have about Yubo or any other social media app.